Understanding Decision Trees
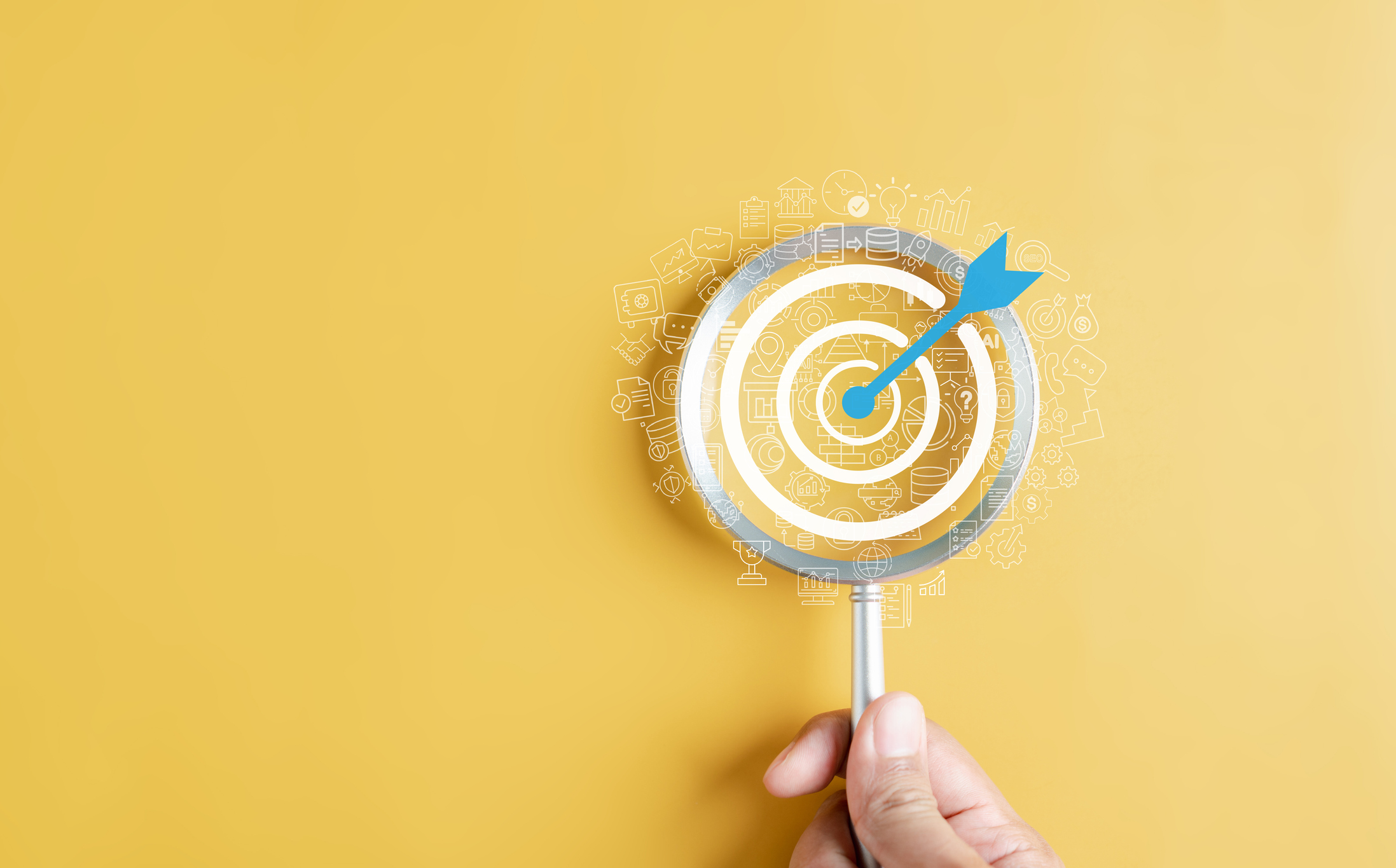
A Powerful Tool for Effective Marketing
Ellipsis Data Scientist, Ana Popovic shares how decision trees can be incorporated to improve marketing and customer loyalty initiatives.
Introduction to Decision Trees
Marketing professionals, business owners, and data analysts continuously seek reliable methods to understand complex customer behaviour in the current data-driven landscape. Decision trees — a powerful predictive modelling tool – can positively impact your marketing strategies. From predicting customer behaviour to targeting specific customer segments, decision trees provide a framework that can dramatically increase the efficiency and effectiveness of your marketing efforts.
How do Decision Trees work?
A decision tree begins with a ‘root node’, representing an entire population or sample, e.g., your customer loyalty base. This node then divides into more similar groups called ‘decision nodes’ using a method called ‘splitting’. Each node in the tree symbolises a customer feature, e.g., age, preferred channel, frequency. The lines that connect nodes are called ‘branches’; these are ‘decision rules’ or questions that help categorise customers into more consistent groups, e.g., “Does this customer prefer online shopping?”. The resulting groups of customers are called ‘leaf nodes’; these nodes do not split any further.
Let’s look at an example decision tree for an online shopping site to understand how all those terms and ideas come together, working through the branches down to leaf node five (bottom right node in the image below). This group of customers is characterised by a preference for Online shopping, an age over 30 years and a purchase frequency over 4.
The decision rule at the root node usually addresses the most significant and general patterns in the data because it’s the starting point for segmentation. Ideally, this initial split should result in a substantial improvement in customer similarity compared to the unsplit data. As you move deeper into the tree, the decision rules tend to address more specific customer patterns or nuances. The aim is to refine the segments and achieve higher similarity between customers.
This data visualisation using the tree-style layout offers an intuitive way for different teams to understand customer behaviours and improve clarity in decision-making. Decision trees employ a ‘divide and conquer’ strategy. They search for optimal ways to group customers and keep doing this top-down until most customers fit into distinct categories. However, as these trees expand, maintaining pure groups becomes challenging. Overly large trees might lead to ‘overfitting’—making too specific assumptions and resulting in very small customer segments in the leaf nodes. Hence, simpler trees are often preferred for more precise insights. To prevent overfitting, ‘pruning’ is employed, trimming away branches based on less relevant features. The tree’s effectiveness is then assessed using ‘cross-validation’, ensuring it remains straightforward yet insightful for fine-tuned marketing strategies.
Predictive Modelling with Decision Trees
For predictive modelling, decision trees provide a structured way to visualise the results of each decision node and a very flexible approach to creating that structure, allowing many data types (e.g., total purchases and gender) to be used for splitting.
By inputting customer data—such as transactional data, interactions with marketing campaigns, and socio-demographic characteristics—decision trees can help predict a range of customer behaviours. One such behaviour of significant importance to businesses is customer churn. For instance, data for the online shopping site revealed that customers who sign up are significantly less likely to churn and that those who have shopped across multiple categories have a decreased churn rate. Moreover, purchasing a specific product further reduced the likelihood of churn, highlighting the nuances that decision trees can uncover.
Customer churn is a crucial element in any business, and decision trees can help predict which customers are likely to become frequent and active buyers and which are prone to attrition. This form of predictive modelling can save businesses considerable resources. Instead of employing a blanket approach in customer retention efforts, companies can focus on segments more likely to bring long-term value, maximising Return on Loyalty®.
Targeting Customer Segments with Decision Trees
The effectiveness of any marketing strategy hinges on its ability to target the right audience. Here, decision trees come into play as powerful segmentation tools. By analysing large datasets, they can identify meaningful patterns and correlations that could go unnoticed.
Drawing from the earlier example involving the online shopping site decision trees highlight customers who are less likely to churn and identify potential risk segments. In this case, customers who did not sign up and did not purchase a specific product showed a significantly higher likelihood of churn. With this detailed breakdown, businesses can craft tailored marketing strategies that resonate with specific customer segments, increasing the likelihood of conversions and customer engagement.
Optimising Marketing Efforts with Decision Trees
Marketing is an ever-evolving field that demands adaptability and a constant revaluation of strategies. Decision trees are naturally equipped to assist with this kind of optimisation. Unlike other predictive models that are static and awkward to update, decision trees can easily be adjusted with new data and insights.
Suppose a particular marketing campaign fails to generate the expected results. In that case, a decision tree can help pinpoint the likely causes—the wrong target audience, ineffective communication channels, or poorly timed execution. By continually updating the decision tree model, businesses can achieve a dynamic form of marketing optimisation, ensuring that strategies remain effective amidst changing market conditions and customer behaviours.
Key Takeaway
Understanding decision trees is vital for marketing professionals, business owners, and data analysts who aspire to excel in today’s data-driven business landscape. With their ability to deliver insightful predictive modelling, precise customer segmentation, and dynamic marketing optimisation, decision trees are invaluable for crafting effective and efficient marketing campaigns.
By integrating decision trees into your marketing efforts, you adopt a sophisticated analytical tool and a mindset focused on continuous improvement and customer-centricity. In a marketplace that is more competitive than ever, decision trees can give you the critical edge needed to understand your customers better, serve them more effectively, and ultimately succeed in your business endeavours. Interested in learning more or seeing decision trees in action? Please reach out to our team to organise a chat.
We are Ellipsis, the Loyalty Experts. We help you measure, manage and grow customer loyalty. We’re here to help, please get in touch…